How to boost OTT engagement with personalized recommendations
- AI
- Recommendations
In the competitive landscape of Over-The-Top (OTT) platforms, personalized recommendations are crucial for enhancing user engagement and retention.
Whether you aim at building or improving your OTT platform, understanding and implementing effective recommendation algorithms becomes vital.
Personalized recommendations not only improve user experience but also drive content discoverability and platform loyalty.
But how exactly do these recommendation systems work, and what benefits and challenges do they present?
Personalized recommendations are generated by sophisticated algorithms using artificial intelligence (AI) and machine learning (ML) to analyze vast amounts of user data. The goal is to suggest content tailored to individual preferences, thus enhancing the overall user experience. These algorithms consider multiple data points and behaviors to make accurate suggestions.
Key components include:
- User profiling: Collecting and analyzing data on user demographics, preferences, and viewing habits.
- Content analysis: Evaluating the metadata of available content, including genres, actors, and keywords.
- Behavioral patterns: Studying user interactions such as watch history, ratings, searches, and browsing patterns.
- Feedback loop: Continuously updating the recommendation model based on user feedback and new data.
The combination of these components ensures that the recommendations are as relevant and engaging as possible, thereby increasing user satisfaction and platform usage.
Personalized recommendations offer several significant benefits that enhance the performance and appeal of OTT platforms.
- Enhanced user experience: Personalized recommendations provide content that aligns with users' interests, making the viewing experience more enjoyable and engaging.
- Increased user loyalty: By keeping users returning to the platform, personalized recommendations help reduce churn rates.
- Reduced editorial efforts: Automating content recommendations decreases the need for manual editorial intervention, allowing for more efficient operations.
Netflix exemplifies the success of effective personalized recommendations. The platform uses a combination of content-based and collaborative filtering methods, continuously refined through user feedback and extensive data analysis.
This approach has been instrumental in Netflix’s success, with personalized recommendations accounting for over 80% of the content watched on the platform. Netflix's ability to suggest relevant content keeps users engaged and increases their viewing time, contributing significantly to user satisfaction and loyalty.
Video streaming platforms collect and utilize extensive data, which can be categorized into:
- Video metadata: Information such as titles, descriptions, genres, and images that describe the content.
- User data: Includes demographic details, viewing devices, and geographic locations.
- Interaction data: Covers users' viewing history, purchases, navigation patterns, favorites, and social shares.
This data is essential for creating enriched metadata and accurately understanding user preferences. Continuous analysis and updating of these data points ensure that the recommendation algorithms can provide relevant and personalized content suggestions.
1. Content-based filtering:
This method analyzes the content a user has interacted with to suggest similar items. For example, if a user frequently watches science fiction movies, the algorithm will recommend more sci-fi films. Categories like "Associated content”, "Because you just watched”, and "For you" are typical examples of content-based recommendations, ensuring that the suggestions are directly relevant to the user's past behavior.
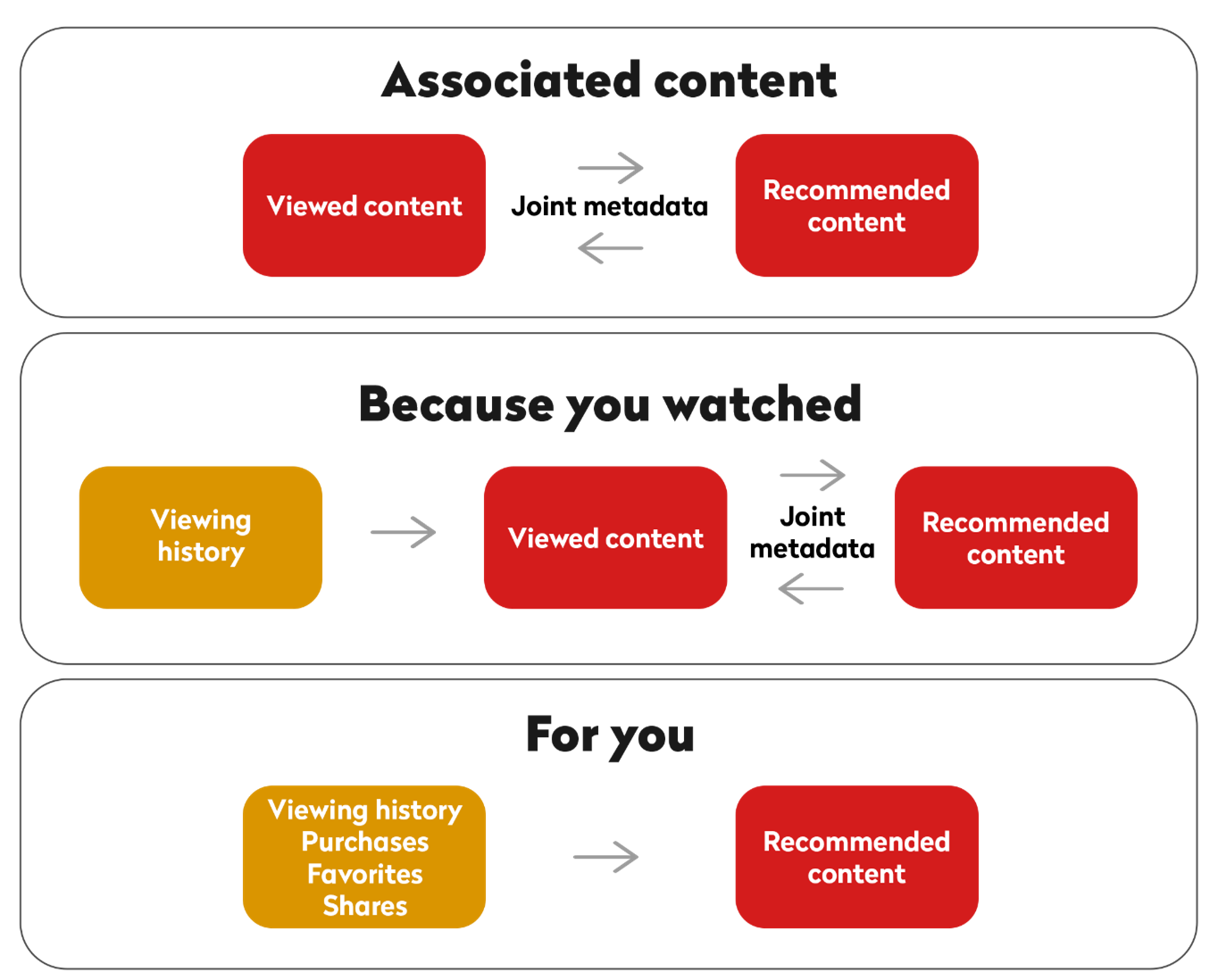
2. Collaborative filtering:
This technique uses the preferences and behaviors of similar users to make recommendations. For instance, if users who watched a particular movie also enjoyed a specific TV series, the system will suggest that series to others who watched the movie. Categories like "Most popular” are examples of collaborative filtering, leveraging the collective behavior of the user base to provide personalized suggestions.
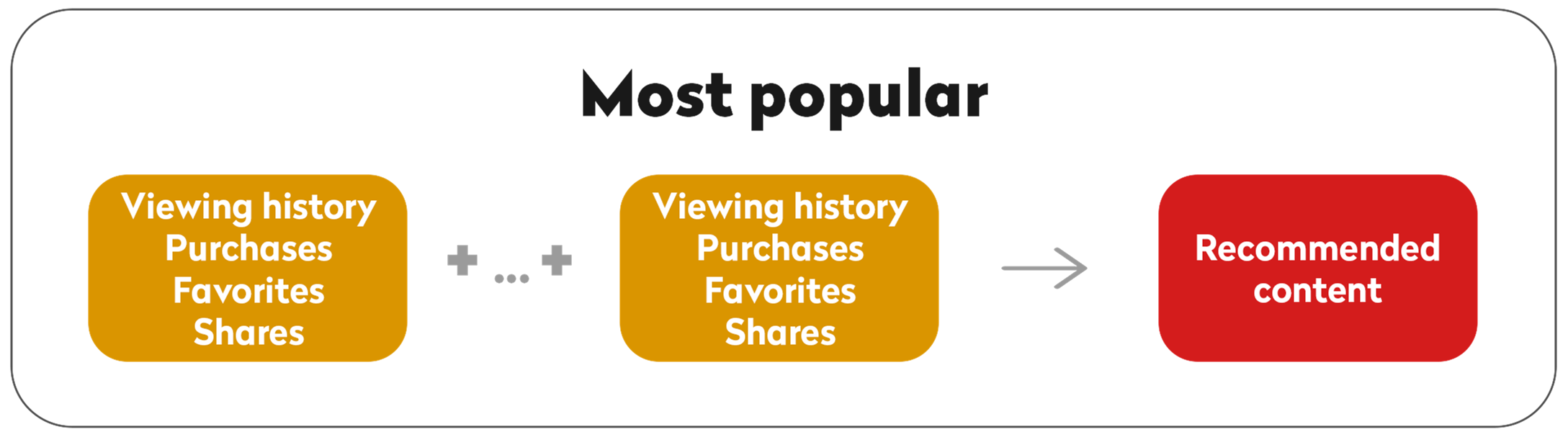
Effective recommendation engines significantly enhance content discoverability on OTT platforms. By suggesting relevant yet potentially overlooked content, these systems encourage users to explore more of the platform’s offerings. This personalized approach not only keeps the audience engaged but also maximizes the utilization of the platform’s content library.
By guiding users to diverse content, these engines ensure that a wider variety of shows and movies are consumed, leading to increased user satisfaction. This comprehensive use of the content library helps reduce churn rates, as users are less likely to seek alternative services when their current platform consistently meets their entertainment needs.
Overall, the strategic implementation of personalized recommendations boosts user retention, engagement, and satisfaction, making it a crucial feature for any successful OTT service.
1. Relevance vs. serendipity:
Balancing between suggesting content that users are likely to enjoy (relevance) and introducing them to new, unexpected content (serendipity) is a key challenge. Implementing algorithms that mix predictable recommendations with occasional novel suggestions helps maintain user interest and avoids content fatigue.
2. Data quality:
Ensuring high-quality, well-structured metadata is essential for accurate recommendations. Continuous improvement of data collection processes and investing in metadata enrichment tools are vital practices for maintaining data quality and ensuring the effectiveness of recommendation systems.
3. Transparency and user control:
Users may feel overwhelmed or distrustful of automated recommendations, if they lack transparency. Providing users with control over their recommendation profiles, allowing them to adjust preferences and rate content, enhances the system’s accuracy and builds trust. This user feedback loop is crucial for refining and personalizing the recommendations.
The OTT landscape is dynamic, and user expectations are constantly evolving. To stay ahead of the curve, personalized recommendations are no longer a luxury, but a necessity. By leveraging the power of AI and user data, streaming platforms can create a hyper-personalized experience that keeps users engaged, reduces churn, and fosters loyalty.
However, building and maintaining effective recommendation systems requires expertise and cutting-edge technology. This is where Alpha Networks steps in. Our personalized recommendation features, part of our advanced end-to-end OTT platform Gecko, empower OTT platforms to deliver exceptional user experiences.
Contact us today to unlock the full potential of your video platform.
Alpha Networks will be attending the upcoming SET Expo 2024! This event is the premier trade show for the broadcast and multimedia...
Read publicationAlpha Networks, a leading provider in hybrid TV back-ends & smart video platforms, today announced that Bouygues Telecom launched...
Read publicationHares & Co., a major media and entertainment consultancy specialising in content distribution and monetization through digital pla...
Read publicationPréparez-vous à sublimer vos contenus avec des produits d'exception.
Prenez le contrôle de votre contenu et partagez-le avec le monde. Faites partie de la révolution télévisuelle dès aujourd'hui.
Parlez à un expert